The DNA of better trip matrices
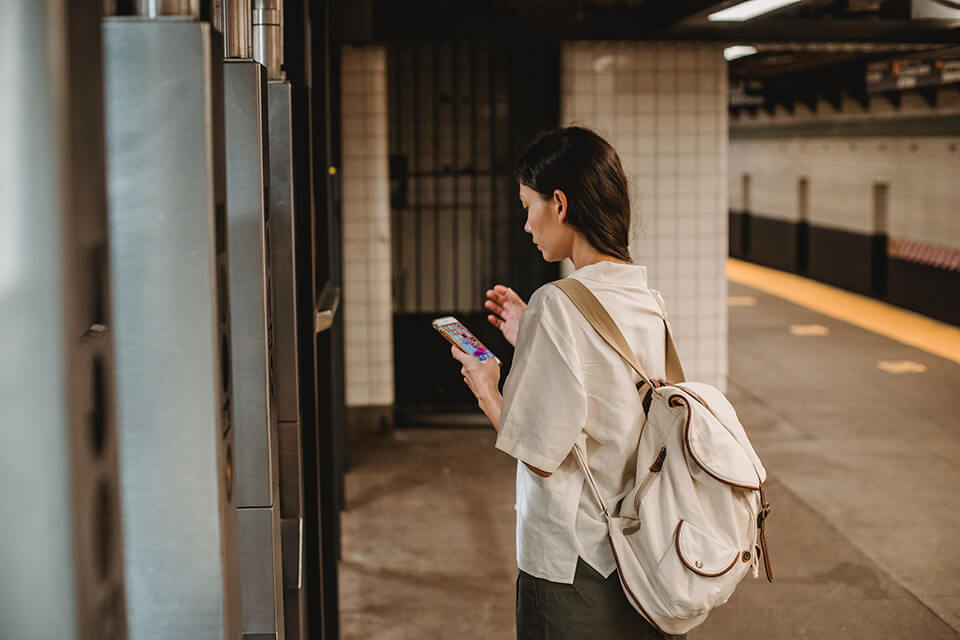
Not all mobile phone trip matrices are born the same [Part 1]
The traditional travel data collection methods have proven expensive, disruptive and limited, but new technologies have recently allowed to generate mobile phone trip matrices that could potentially overcome many of these constraints as they can provide door-to-door movements based on a device that most people carry with them at all times. So, how does this work?
The use of trip matrices based on mobile phone data has become a practical proposition in many countries. They are frequently used in the US from sources such as AirSage and StreetLight. They are also widely used in Spain and the UK from sources such as Telefónica and our Nommon company. Other countries currently explore their reliability for practical use replacing at least some of the most expensive forms of travel data collection.
There are some good reasons for this interest in mobile phone trip matrices. They offer the potential to overcome some of the limitations of current travel data collection methods at a reasonable cost.
Travel data collection methods
As we know from practice, the travel data collection methods we have been using for the last 30 years have important limitations. Household Travel Surveys offer the richest source of trip making and choice insights. However, they are very expensive to collect thus limiting sample sizes to 1% or 2% of the population. Moreover, they are disruptive and disliked by households who seem to resent the intrusion and length of the survey.
The collection of more than one day travel diary is difficult and respondents are prone to simplifying answers to reduce the burden; this results in a universal under-reporting of trips that must be corrected by other means later in the process. Intercept surveys (roadside and person interviews) permit larger samples but are increasingly difficult to undertake, as people dislike the disruption to their journeys. Stated Preference/Choice surveys suffer from a similar set of limitations plus the difficulty in ensuring the realism of the responses. Number Plate Surveys are useful but they only provide information for vehicle movements on a local (from camera to camera locations) level. Luckily, two essential forms of data collection are non-disruptive and easier to collect in larger samples: Traffic Counts and Travel Time Surveys.
The limitations of conventional data collection techniques result in imperfect information for an “average day” (in reality a mix of observations on different days) with plenty of empty cells in the trip matrix without knowing whether this is due to sampling or a real absence of trips.
New technologies
More recently, new data collection techniques that have become available offer larger sample sizes and longer timespan coverage: Bluetooth and WiFi surveys and the processing of public transport smart cards and mobile phone records. These are all passive, non-disruptive forms of data collection. Bluetooth and WiFi surveys pick up data mostly from mobile phones but suffer the same limitation as Number Plate Surveys as only local movements are collected. Smart card data again covers personal movements from stop to stop but in many cases only the boarding is identified, the alighting location has to be estimated from other movements.
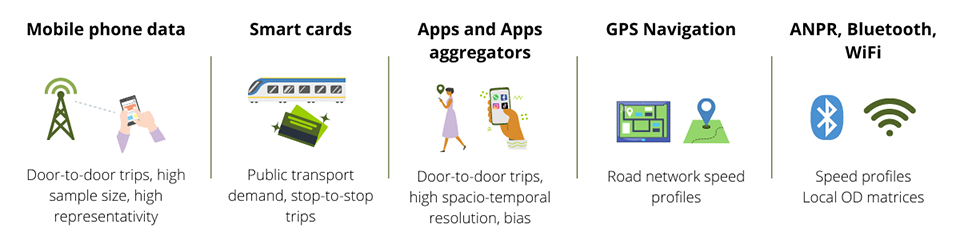
The generation of mobile phone trip matrices could potentially overcome many of these limitations as it can provide door-to-door movements based on a device that most people carry with them at all times.
There are at least two types of suppliers for mobile phone trip matrices. One is the Mobile Network Operator (MNO) itself, seeking to monetise directly the data they have from transactions (Call Detail Records or CDRs) and cell location and coverage (Cell Map). Sometimes consultants take these MNO trip matrices and further adapt them to fit more closely the needs of end-users. Second, there are a few data analytics companies like Citi Logik, Teralytics and Nommon; they process the raw data (CDRs and cell maps) from MNOs and deliver trip matrices and other mobility indicators directly adapted to the requirements of the final user.
Despite its relative popularity and the range of suppliers, the acceptance of mobile phone generated trip matrices has not been universal. There are many stories of frustrated users disappointed by the quality of the mobile phone trip matrices they get. They criticise the realism of the matrices produced in this way and some of the inherent limitations of the technique: for example it is not impossible to distinguish the mode of transport used in dense urban areas. On the other hand, some clients seem to be very happy using them, and they come back (at least to us) for more when the occasion arises. Why is this the case? What makes some trip matrices better than others for some particular applications?
Why the difference?
We do not know the full answer to these questions. After all, the basic algorithms to extract trip matrices from mobile phone data are published and available to whoever has data analytics, programming skills and resources to implement them. So, the problem is not the basic techniques necessary to produce the trip matrices.
One of the causes for disappointment is obviously the hype surrounding many of the offerings related to these new data sources. Mobile phone trip matrices have been oversold as the solution to all the travel data collection needs and woes. Sadly, this is not the case. They are a useful addition to the mobility data sources but they are unlikely to replace all currently collected data. Reliable classified traffic counts are likely to be needed for many years to come. Household Travel Surveys (HTS) offer a rich and irreplaceable source of data, for example on car ownership and user preferences. However, a smaller (cheaper and more reliable) sample of household surveys would now suffice when combined with mobile phone data. Stated Choice surveys also offer valuable insights into travel preferences and behaviour that would be difficult to replace with mobile phone data today (although some progress has been achieved in respect of the willingness to pay to save time and this may extend to other parameters in the future).
In addition, it must be recognised that mobile phone data also has important limitations as a data collection method. For example, unless the circumstances are exceptional, mobile phone data is not accurate enough for travel time estimation, therefore other GPS based data sources are to be preferred. Although it is generally feasible to estimate mode and route with mobile phone data in the case of inter-urban travel, this is much more difficult -and we would not recommend it- in dense urban areas; in those cases data fusion approaches are necessary.
Read “The nature of mobile phone data: Not all mobile phone trip matrices are born the same [Part 2]“