Transport models and mobile phone data
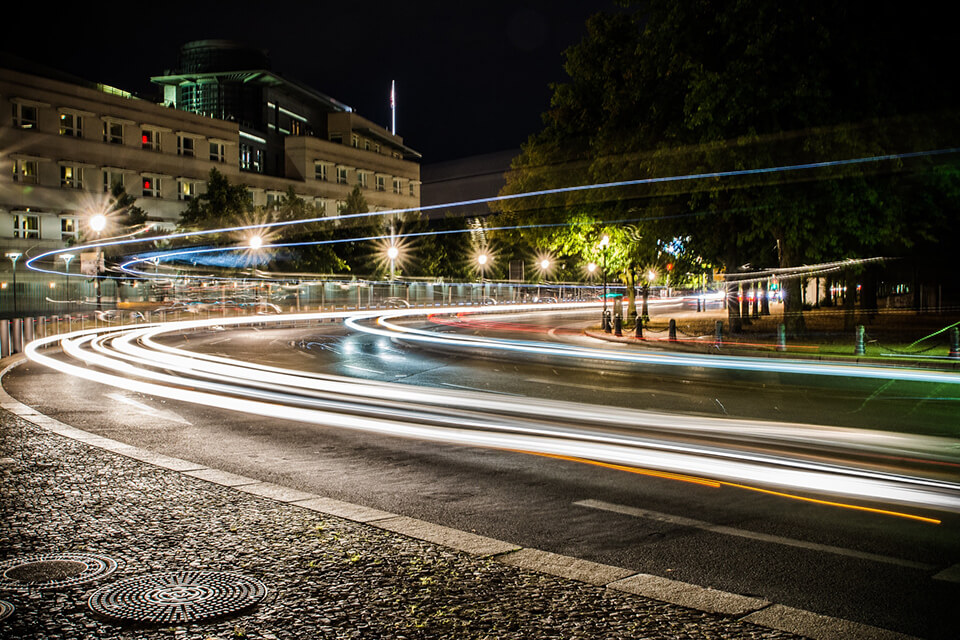
Let’s have a look first at the use of mobile phone data on our existing models. We have been operating very consistent types of transport models for the last 50 years with some more recent modest innovations. Classic transport models can operate at different levels of analysis: aggregate and disaggregate trips, tours and activities; all of these are extractable from mobile phone data. The most common type of transport model is the aggregate kind, often based on trips. The main limitation of trip based models is that many choices, for example choices of mode, are not independent: the mode used on trips back home, for example, by owned car, depends on the mode used when leaving home.
Current best practice recommends that trips should be handled in one format for demand models and a different one for assignment. When developing demand models, the work on matrices should be done first at the Production/Attraction (P/A) form and then converted into origin-destination matrices for Assignment. The P/A format is equivalent to a From and Back Home trip purposes naturally obtained from mobile phone data. This P/A arrangement is considered a better basis for modelling mode and destination choice as they incorporate the characteristics of at least two essential legs of any tour.
An additional enhancement to transport models would be to treat tours rather than simple trips. This is a bit more complex than dealing with the P/A structure and requires identifying representative tours. Again, mobile phone data is well placed to deliver these with a much larger sample rate than using a Household Travel Survey. The main limitation, of course, is that only simpler tour stop purposes can be identified from this source alone.
The set of activities obtainable from mobile phone data alone is, as yet, very simple and enhancing it requires fusion with other data sources. Nevertheless, mobile phone data naturally link activities providing a rich set of tours to select the most significant for modelling. The identification of mode of travel should be possible, in the first instance, using other data sources like public transport smart cards and person and classified traffic counts. The precise way to achieve this will depend on the availability of this additional data.
Finally, some refinement will be possible during the calibration process of the model itself.
All of these transport modelling efforts could be undertaken at an aggregate or disaggregate behavioural level. Therefore, it is possible to use mobile phone data to implement a range of modelling improvements following classic lines and using existing complementary data sources. However, we know now that the future presents challenges that are difficult to represent at an aggregate location level.
The future of modelling
There are three main mobility challenges that future transport modelling must address: Electric Vehicles (EV), Mobility as a Service (MaaS) and Connected and Automated Vehicles (CAVs). EVs behave in traffic like ICE vehicles but need charging points at specific locations; for instance, this is critical for a good EV taxi service. The optimal location of these charging points depends on their patterns of use and can still be estimated using aggregate models.
This is not the case for MaaS and CAVs. Autonomous Vehicles (Levels 4 and 5) may travel empty to serve other members of the family and friends or join and be part of a MaaS fleet; in modelling terms this requires tracking not just passengers but also vehicles. MaaS adds another dimension to tracking vehicles: fleet management to serve customers at specific locations that may or may not wish to share rides with others entailing efforts to optimise routes. Estimating the efficiency of this management, the level of service provided to users and the impact on congestion and emissions requires identifying individual timed requests and how well they are served with a particular arrangement and fleet.
Attempting to model these aspects with aggregate models can only be done as a very coarse approximation. A serious treatment of these features practically requires an agent-based micro-simulation approach tracking individual vehicles and trips between activities and how best to attend requests at specific locations, not centroid connectors. This has been modelled, for example, by the International Transport Forum, modelling MaaS for Lisbon, Auckland, Dublin and Helsinki. To populate their agent-based models ITF expanded the ~2% sample of the population from the Household Travel Surveys creating a synthetic population with specific (time and location) requests for trips and modelling the supply of ride-sharing services to serve them.
In our view at Nommon, this type of approach can also be assisted with mobile phone data and achieve even more realistic results. We explored these approaches with PTV using their MaaS Modeller in Barcelona as part of the CARNET initiative. In this case Nommon provided aggregate trip matrices and these were then used to create a synthetic travelling population requesting trips at specific locations and times. MaaS Modeller then organises the fleet available to satisfy this individual demand with ride-sharing and other services and calculates key performance indicators for fleet managers, users and the community.
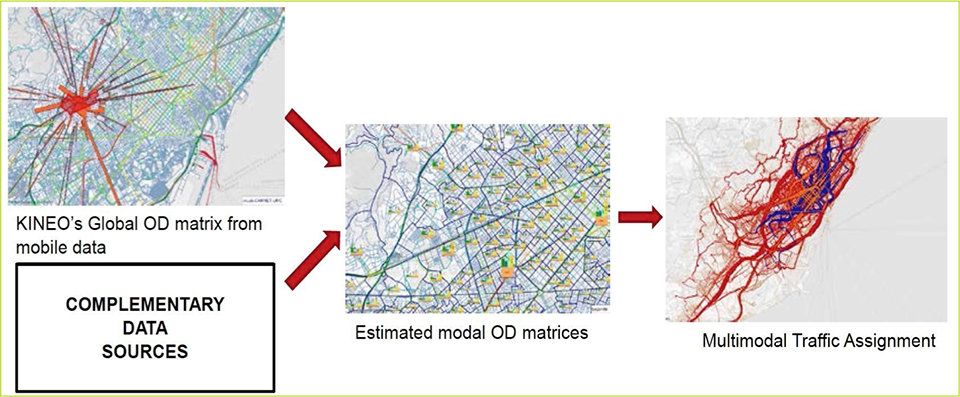
Agent based modelling
It is possible to use mobile phone data even more efficiently. The basic outcome of our processing of the mobile phone records is a set of activity-travel diaries for a real population. The next step would be to set up a full artificial population that mimics the total population under study. To generate this, we start from the activity-travel diaries of the mobile phone users and use census data to expand the sample by means of classical synthetic population techniques. In that way the new agents added to expand the sample are also assigned properties like age, gender, etc.). In many projects these individual trips are later on aggregated to generate origin-destination matrices. However, especially when the purpose is to feed disaggregate models, direct use of individual anonymous activity-travel diaries would indeed enable much richer analyses and open the door to more interesting modelling approaches.
We have done this in research projects, for example to feed an agent based activity[1] model to study cordon toll policies and we also plan to explore the benefits of using these individual diaries in some of our ongoing joint research projects with Aimsun. The idea is that the timing and (x, y) coordinates of each activity in each individual diary of the total synthetic population are randomly allocated within the coverage area of the corresponding cell (based on probabilities that take into account land use data, the population density of the different census tracts intersecting that particular cell, etc.). This should not be considered as personal information anymore, as no travel diary corresponds to the actual profile and the exact coordinates of any real person.
An obvious limitation is the number of different activities that mobile phone data can identify. The most important (and recurrent) ones of Home, Work, Study are easy but others are not; therefore, opportunities for combining other activities in new ways in the future will be limited. However, new complementary data sources (for example weblogs and pico-cell data) are expanding the range of activities that can be identified and therefore this limitation may be relaxed in the future.
On the other hand, we do know a couple of things about activities that tend to reduce the importance of differentiating a large number of activities. First, activities are not the same day-after-day, that is they are not that recurrent. We do know that at most half of the trips on a network at any one time can be considered to be recurring. Second, many activities do not remain the same over the years. Examples that come to mind are video rentals, supermarket shopping, gym visits and video conferencing. These two characteristics of activities put in question to what extent we can reliably forecast them into future years.
Learning from quasi-experiments
Some knowledge domains benefit from plenty of opportunities to do experiments and learn from them, for example, agriculture or metallurgy. Because of the cost and disruption that experimentation entails this is seldom possible in the field of transport. Given that mobile phone data provides a continuous stream of data to monitor movements this creates an interesting opportunity to learn from experience, in particular from planned and unplanned quasi-experiments. For example, monitoring what people do when a metro line is closed for a day or a longer period should be illuminating in terms of preferences and adaptation of travel patterns. Bad weather could disrupt travel and force trip makers to change routes, modes and even destinations and research on these adaptations could result in improved and empirically supported choice models.
Mobile phone data thus provides new and rich openings for further research into travel behaviour and transport models that may remove the reliance on Stated Preferences/Choice methods and provide a more solid ground for practical applications and more reliable forecasting.
Adapting to change
A rapidly evolving future places additional demands on planners and decision makers. It requires a more rapid updating of models and a more sensitive detection of changing trends and technology disruptors. Moreover, the work environment is becoming more flexible and responsive to changes in behaviour and this creates more variable trip making patterns; this may well require the analysis of travel on different days rather than on the mythical “average and neutral” one. The usual ten years between Household Travel Surveys diminishes their value as a trend monitoring tool. The greater variety of mobility options, from walking and micro-mobility to Demand Responsive Transport and shared rides requires much larger samples than feasible with conventional data collection methods.
All of these influences point to the use of passive data collection methods that rely on traces naturally generated as part of our daily life. Anonymised mobile phone data with suitable measures to protect privacy and algorithms refined in practice appear to be an ideal source of data to address the challenges of a rapidly evolving mobility landscape.
In summary, data from mobile devices has limitations, most of which can be overcome with better algorithms and intelligent data fusion. On the other hand, mobile data will not just help to reduce costs and delays of travel data collection. It is also creating opportunities for new forms of modelling and monitoring trends to tackle some of the challenges posed by new mobility, including MaaS, CAVs and EVs. Moreover, research into new demand models can now be more solidly grounded thanks to the insights provided by this new data source.
[1] Aleix Bassolas, José J. Ramasco, Ricardo Herranz, Oliva G. Cantú-Ros, “Mobile phone records to feed activity-based travel demand models: MATSim for studying a cordon toll policy in Barcelona“, Transportation Research Part A: Policy and Practice, Volume 121, 2019, Pages 56-74, ISSN 0965-8564, https://doi.org/10.1016/j.tra.2018.12.024.