- Passenger-centric Big Data Sources for Socioeconomic and Behavioural Research in ATM
- Consortium: Nommon (project coordinator), IFISC, Fraunhofer-IAIS, the Hebrew University of Jerusalem, and ISDEFE.
- 2016 – 2018
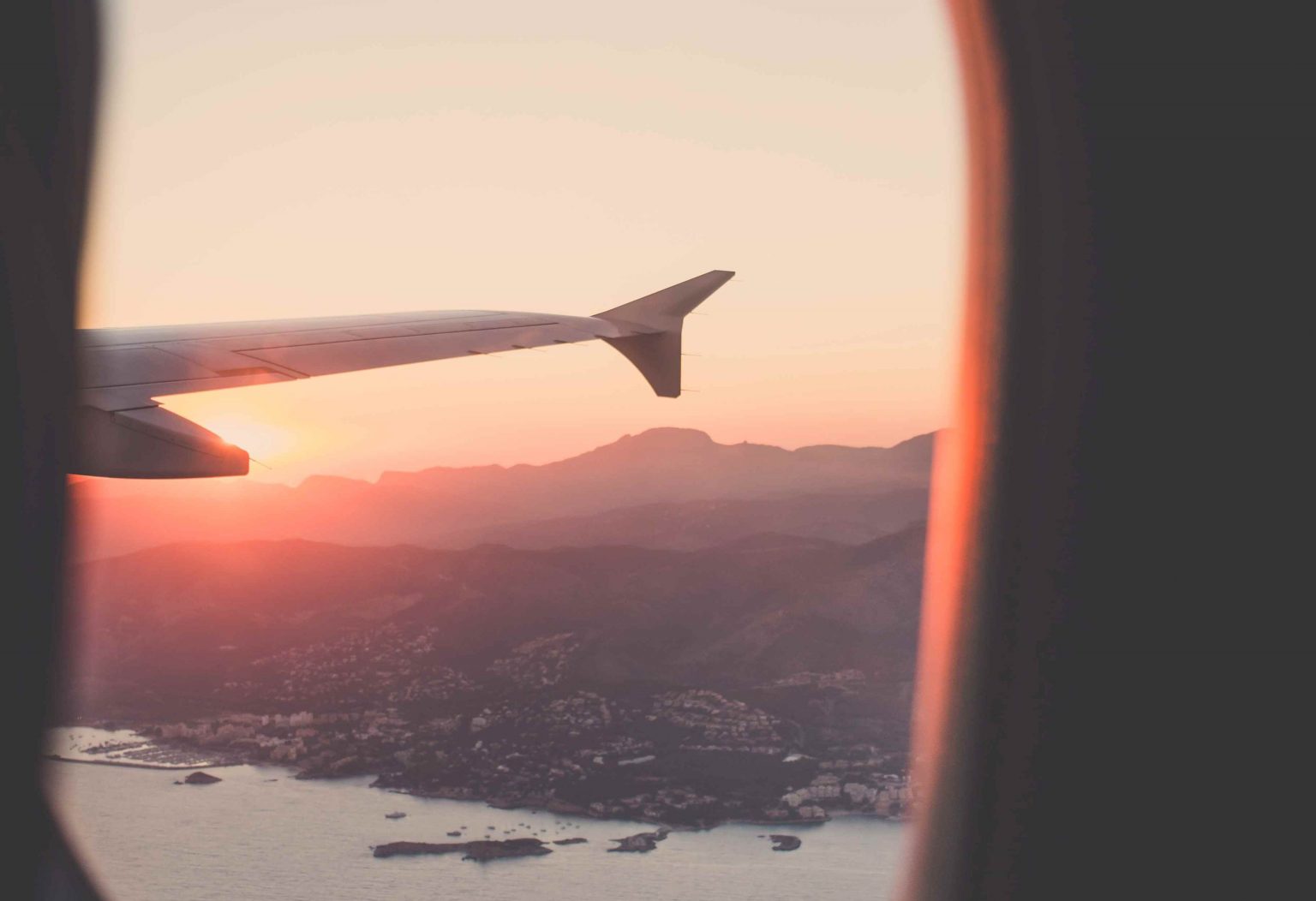
The project
BigData4ATM is a research project within SESAR 2020 Exploratory Research. Its aim was to investigate how new sources of passenger-centric data from smart personal devices can be analysed to extract relevant information about passengers’ behaviour. The goal was to understand how this information can be utilised to inform decision-making processes in air traffic management (ATM). The project was undertaken by a consortium consisting of Nommon (project coordinator); two world-leading research centres in complexity science and data science, IFISC and Fraunhofer-IAIS; a top university in transport economics, the Hebrew University of Jerusalem; and ISDEFE, a consulting company with extensive knowledge of the ATM sector.
Context
The paramount objective of the European transport policy — as outlined in the European Commission’s 2011 White Paper on Transport — is to “establish a system that underpins European economic progress, enhances competitiveness and offers high-quality mobility services while using resources more efficiently.” In line with these goals, the long-term vision for the European aviation sector, as outlined in the report ‘Flightpath 2050 – Europe’s Vision for Aviation’, envisions a passenger-centric air transport system seamlessly integrated with other modes of transportation. The ultimate goal is to provide predictable and efficient door-to-door travel while improving passenger experience and enhancing the system’s resilience against disruptions.
In contrast to this overarching vision, ATM operations have traditionally lacked a passenger-oriented perspective, with performance objectives and decision criteria (e.g., flight prioritisation rules) not necessarily taking into account the ultimate consequences for the passenger. Further research is needed to provide new insights on the interactions between the ATM system and passengers’ needs, choices and behaviour. However, traditional methods for collecting data on passengers’ activities, such as surveys, suffer from limitations in accuracy and validity (e.g., incorrect and imprecise responses, dependence on respondents’ availability and willingness to participate). These methods are also resource-intensive. While data from sources such as air traffic databases, travel reservation systems, and market intelligence services can mitigate some of these limitations , they often fail to capture critical information, such as door-to-door origin-destination pairs and travel times. The widespread use of geolocated devices in daily life opens new opportunities to collect comprehensive data and overcome the limitations of traditional approaches. The same ICT tools enabling bidirectional communication with passengers are also enabling the collection of continuously updated information on passengers’ activity and mobility patterns, with an unprecedented level of detail.
BigData4ATM’s goals
The goal of BigData4ATM was to investigate how various passenger-centric geolocated data could be analysed and combined with traditional demographic, economic, and air transport databases to extract pertinent information about passengers’ behaviour. The project aimed to explore how this information can be leveraged to inform decision-making processes in ATM. The specific objective of the project were:
- To develop methodologies and algorithms for acquiring, integrating, and analysing multiple distributed sources of non-conventional ICT-based spatio-temporal data — including mobile network data, indoor geolocation data, credit card records, and data from internet social networks, among others — in order to characterise passengers’ behavioural patterns.
- To create new theoretical models that translate these behavioural patterns into relevant and actionable indicators for planning and managing the ATM system.
- To assess the potential applications of the new data sources, data analytics techniques, and theoretical models through several case studies relevant to the European ATM system, including the development of passenger-centric door-to-door delay metrics, the improvement of air traffic forecasting models, the analysis of intra-airport passenger behaviour and its impact on ATM, and the assessment of the socioeconomic effects of ATM disruptions.
For more information about the project, you can download the BigData4ATM Position Paper.
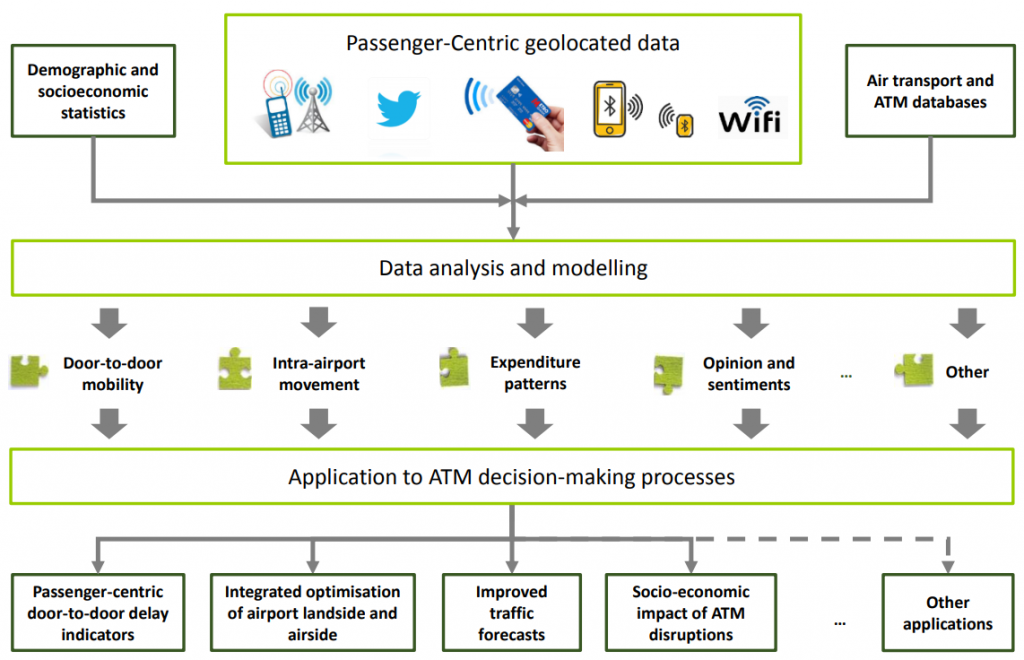
Public deliverables
- D1.3 Final Project Results Report.
- D2.1 Inventory and Quality Assessment of Data Sources for ATM Socioeconomic and Behavioural Studies.
- D3.1 Analysis of Passenger Behaviour from ICT-based Geolocation Data.
- D4.1 Applications of Passenger-Centric Geolocation Data to the Planning and Management of the ATM System: Case Studies.
Scientific papers
- P. García, O.G. Cantú Ros, C. Ciruelos and R.Herranz (2017) “Understanding Door-to-Door Travel Times from Opportunistically Collected Mobile Phone Records: A Case Study of Spanish Airports“, in D. Schaefer (Ed.) Proceedings of the SESAR Innovation Days 2017, EUROCONTROL.
- R. Gallotti, M. Fuster and J.J. Ramasco (2017) “New Data Sources to Study Airport Competition“, in D. Schaefer (Ed.) Proceedings of the SESAR Innovation Days 2017, EUROCONTROL.
- P. García, J.J. Ramasco, G. Andrienko, N. Adler, C. Ciruelos and R. Herranz (2016) “Big Data Analytics for a Passenger-Centric ATM System: A Case Study of Door-to-Door Intermodal Passenger Journey Inferred from Mobile Phone Data“, in D. Schaefer (Ed.) Proceedings of the SESAR Innovation Days 2016, EUROCONTROL.
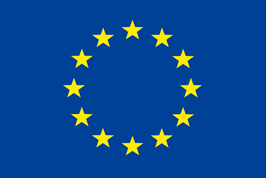
This project received funding from the SESAR JU under grant agreement N.º 699260 under European Union’s Horizon 2020 Research and Innovation Programme.