- Big data platform for the profiling of the passengers and market analysis in smart airports
- Consortium: Nommon, with the collaboration of University of Deusto.
- 2022 – 2023
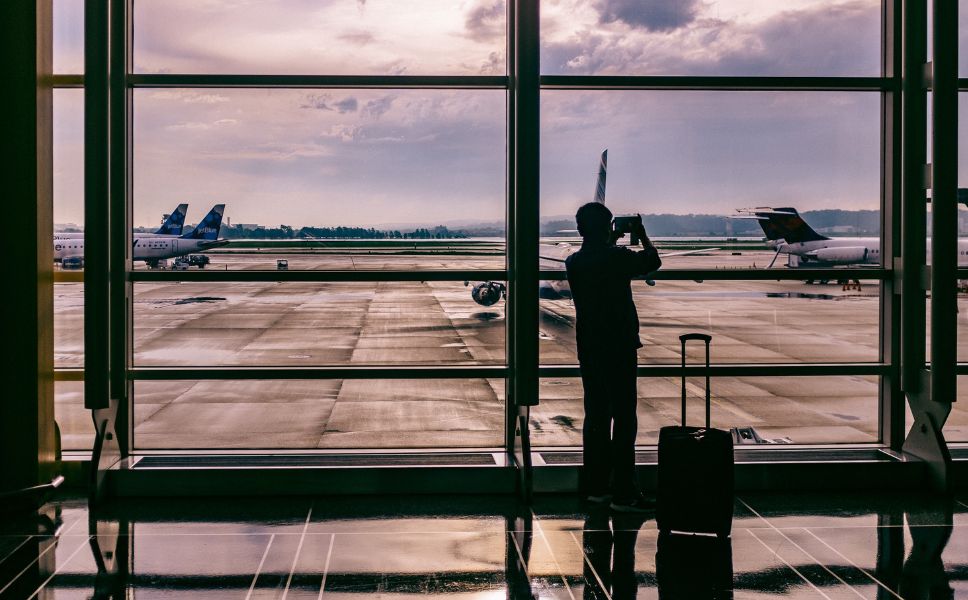
Context
A complete understanding of passenger behaviour, as the final users of the air transport system, is a key aspect for airport planning and management. Airports must be able to adapt to the constantly changing market demand to acquire a better understanding of passenger behaviour and adjust their strategies accordingly. The traditional approach for collecting this information is based on passenger surveys, which have significant limitations in terms of cost, sample size, and update frequency. In recent years, the widespread use of personal mobile devices and IoT sensors has opened new opportunities to collect a vast amount of passenger behaviour and mobility data in a continuous and cost-effective manner, providing a level of detail not achievable with traditional methods.
The project TravelInt
TravelInt is a research project funded by Red.es that proposed to take advantage of these opportunities by exploring how big data and artificial intelligence technologies could be used to enable the detailed analysis of the passengers’ behaviour and support the airport decision-making processes.
TravelInt tackled three specific use cases in which the improvement of the understanding of the users’ behaviour is particularly valuable:
- Route development: this use case analysed and modelled long-distance travel behaviour with the aim of identifying new attractive destinations and offering new routes.
- Accessibility and intermodality: the behaviour of passengers, employees, and other airport visitors in the airport access leg was analysed in order to optimise the connectivity between the airport and its catchment area. The final goal was to improve passenger experience, facilitate the use of more sustainable access modes, and attract potential demand that would otherwise opt for a more accessible airport or an alternative long-distance mode.
- Non-aeronautical revenue: the detailed characterisation of the passengers behaviour within the airport terminal was studied to help optimise the passenger experience and adapt the shopping area and other airport services to the passengers’ preferences and needs.
Goals
The specific objectives of TravelInt were the following:
- To identify the opportunities offered by personal mobile devices to improve the understanding of passengers behaviour and other airport users regarding three main aspects:
- Long-distance travel demand behaviour.
- Airport access and egress.
- Behaviour within the airport terminal.
- To develop new data analysis and fusion methodologies that allow the detailed characterisation of the profile and behaviour of passengers and other airport users.
- To develop a set of machine learning predictive models able to forecast passenger behaviour and support what-if analyses of alternative scenarios and management actions.
- To develop a prototype decision support system that integrates the new algorithms and models developed in the project with an interactive dashboard.
- To validate the proposed system through a set of experiments carried out in close collaboration with the final users in order to demonstrate the maturity of the technology and assess its benefits.
Methodology
TravelInt developed a big data platform that supports the airport operator decision-making process. The platform consists on three main components:
- An ETL (Extract, Transform, Load) process that integrates data from heterogeneous data sources and that performs different data pre-processing tasks (cleaning, formatting, etc.) for their subsequent analysis.
- A processing core which consist of:
- A data analysis layer that integrates different algorithms to analyse and characterise the airport users’ profile and behaviour using the different data sources available.
- A layer for the generation and prediction of indicators that are fed by the information generated in the previous layer, and that consists of three different modules, each of them covering the use case of the platform.
- A visualisation tool that allows the analysis of the results and the benchmarking of different scenarios through a visual interface.
The architecture of the proposed platform is presented in the following figure:
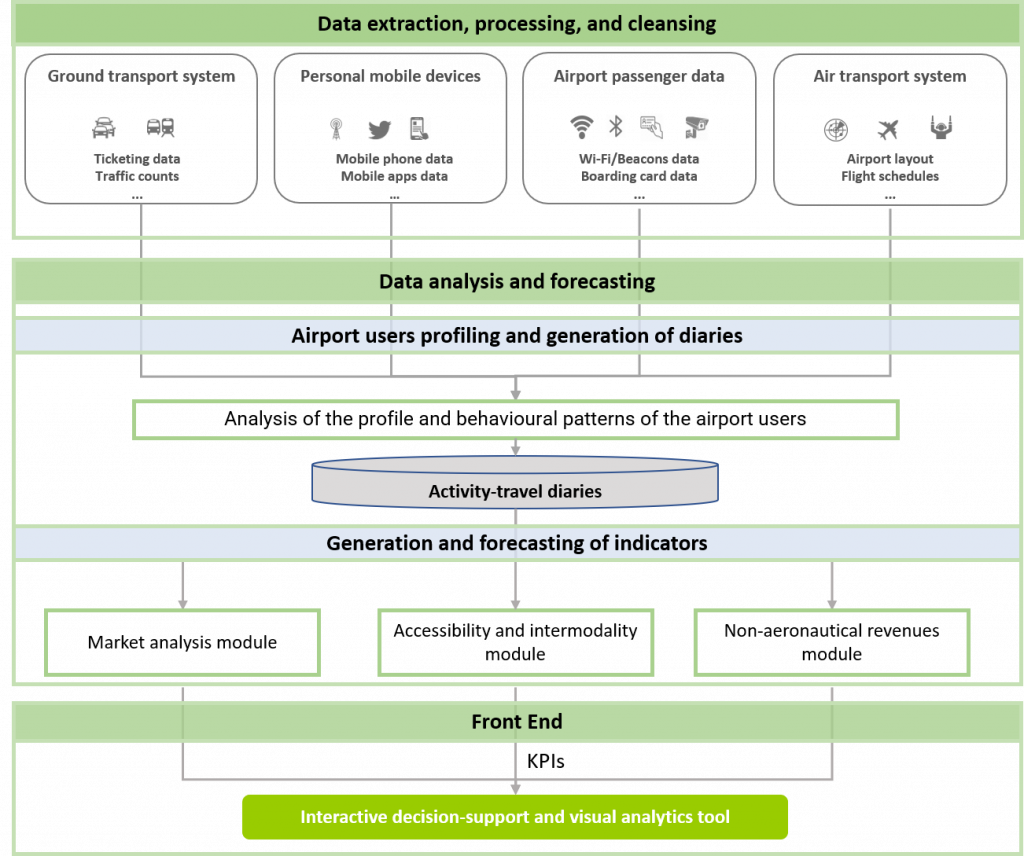
Results
The completion of the project has yielded the following key results:
- It has been demonstrated how data generated by personal mobile devices provide comprehensive insights on the behaviour of passengers and other airport users.
- A set of innovative data analysis and fusion methodologies and techniques have been developed within the project, enabling detailed characterisation of the profile and behaviour of passengers and other airport users with an unprecedented level of precision.
- The detailed characterisation of the historical behaviour allowed the calibration of a set of machine learning models able to anticipate passenger behaviour. These models enable forecasting in different what-if scenarios, facilitating the assessment of the impact of different management actions.
- A prototype of our airport passenger flows management solution WisePax has been developed — an interactive tool that allows the visualisation of historical data, as well as the analysis of what-if scenarios that anticipate passenger behaviour, supporting the final user decision-making process.
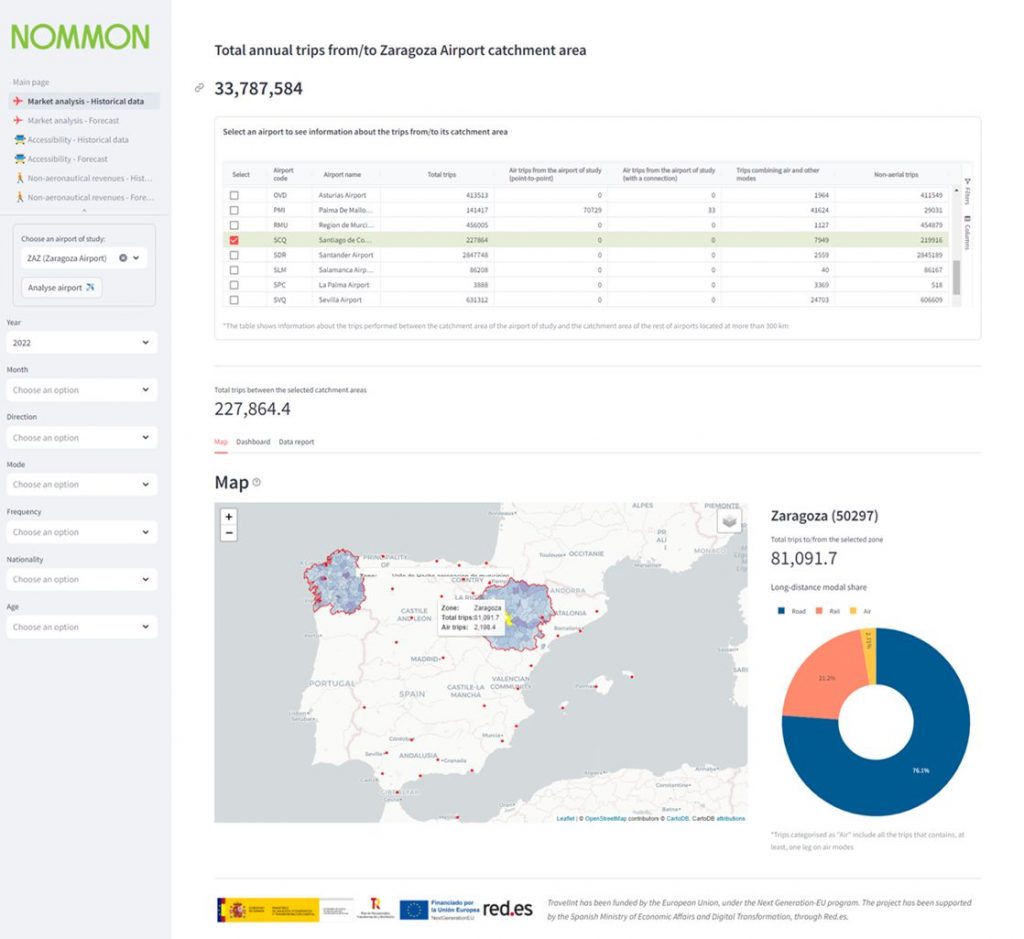
Scientific papers
- Blasco-Puyuelo, J., Burrieza-Galán, J., García-Cantú-Ros, O., Herranz, R., Mocholí, D. (2023). A machine learning approach for predicting airport passenger flows. Proceedings of the 2023 SESAR Innovation Days. https://doi.org/10.61009/SID.2023.1.37
Conference talks and other presentations
- Poster presentation at FlyAI Forum 2023 | Multimodal Collaborative Decision Making based on Advanced Passenger Flow Prediction.
- Presentation at Congreso de Ingeniería y Transporte (CIT) 2023 | Identification of long-distance tour purpose through ML-based data fusion techniques for combining mobile network and survey data.
- Presentation at SESAR Innovation Days 2023 | A machine learning approach for predicting airport passenger flows.
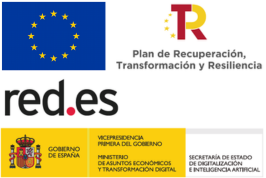
TravelInt been funded by the European Union, under the Next Generation-EU program. The project has been supported by the Spanish Ministry of Economic Affairs and Digital Transformation, through Red.es.