Predicting CO2 savings thanks to shared mobility services in the city of Stavanger
As part of the AI4Cities programme, Nommon and Populus teamed up with the city of Stavanger to develop and implement an AI-driven solution that enables cities to predict shared mobility demand, the modal shift from private cars, and the corresponding CO2 savings, in order to support the design of sustainable mobility policies.
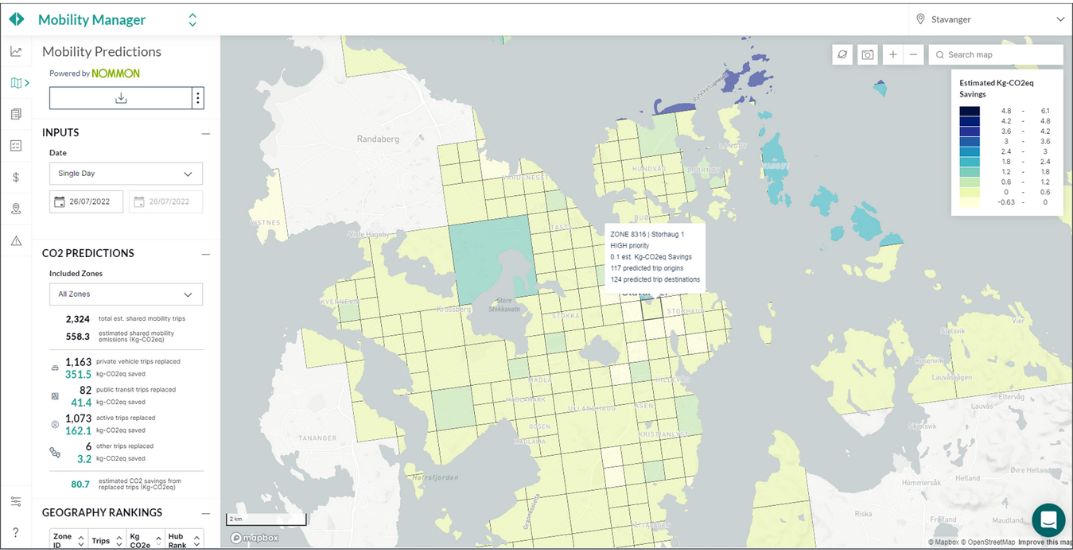
Briefing
Recent years have seen a rapid growth of shared mobility services in cities. Scooters, bicycles, motorcycles, etc. are flooding the streets and promise to be a sustainable solution to replace private vehicles. However, without the right policies and regulations in place, smart mobility may also cannibalise public transport, induce new motorised trips, and compete for public space. As of today, cities still have limited empirical evidence supporting the claim that smart mobility effectively contributes to the much-needed transition towards a more sustainable transport system.
Stavanger, Norway, is one of the 100 cities chosen to participate in the EU Mission for climate-neutral and smart cities. The city is looking for innovative solutions to achieve its objective of becoming climate neutral by 2030. Regarding shared mobility, Stavanger has only recently deployed these new modes of transport and is starting to implement policies and regulations.
Within the AI4Cities programme, Nommon and Populus teamed up with Stavanger in the implementation of AVENUE, an AI-based solution that enables cities to analyse the prediction of expected demand for shared mobility trips and the corresponding CO2 savings in order to design regulations and policies that encourage a modal shift from private cars to more sustainable modes.
Solution
The solution was based on the integration of two tools: Populus Mobility Manager, which allows cities to collect, aggregate, and analyse data from shared mobility operators, and Nommon’s WiseRide, a decision support platform for the planning and operation of shared mobility services that addresses both mobility operators and public authorities. The AVENUE solution included two machine learning models: (i) a regression model that predicts the demand for shared mobility services for each origin-destination pair, and (ii) a classification model that infers the substitution mode that would be used in the absence of shared mobility services. The combination of these models allowed AVENUE not only to predict future shared mobility demand under certain policies but also to predict modal shift, enabling the estimation of the impact of shared mobility services on the city’s carbon footprint. The platform predicts demand for zones currently covered by shared mobility services as well as in locations where such services are not in operation yet, which allowed Stavanger to analyse scenarios of system expansion.
The following figure provides an overview of AVENUE’s technical solution:
AVENUE’s implementation in Stavanger was built upon the data provided by the electric scooter operators Tier and Voi. To validate the AVENUE solution, a comprehensive monitoring of the demand predictions was conducted by comparing them against the observed trips. The graph below shows the demand for scooters predicted by AVENUE for July 2022 and the demand actually observed in the city for the same period. The prediction model accurately captured both the volume and demand trends.
Outcomes
End users in the city of Stavanger were given access to the AVENUE web-based interactive visualisation platform, which integrated the models’ results through an API connection. The tool allowed the users to select a future date and access a set of predictive indicators tailored to their specific interests, as defined during the project’s requirements gathering phase. These indicators included:
- Demand projections for shared mobility trips starting and ending in each of the designated zones on the map.
- Estimated CO2 emissions from shared mobility trips and from each of the substitution modes.
- Estimated CO2 savings due to the predicted modal shift.
The outcomes of the project suggested that by incorporating the policy recommendations provided by AVENUE regarding the new areas of expansion and the location of new mobility hubs, the city could potentially achieve a reduction of 4-5% in CO2 emissions from urban transport by 2025.
What we learnt
Cities face the challenge of developing policies and regulations that maximise the benefits of new mobility services while preventing undesired effects, like the abandonment of public transport. None of these goals can be met without the ability to understand, predict and steer user behaviour. The work carried out in the AVENUE project with the city of Stavanger and the operators Tier and Voi helped us evolve our WiseRide solution to better support policy makers in harnessing the potential of shared mobility services. WiseRide enables cities to fully unlock the potential of shared mobility services by addressing problems such as reducing GHG emissions, as demonstrated in the AVENUE project, but also other challenges associated with environmental sustainability (e.g., local air quality, noise, energy consumption) and operational efficiency, such as optimising the location of mobility hubs’ and making system expansion decisions.